跨過這些坎,人工智能將為全球經濟貢獻14萬億美元
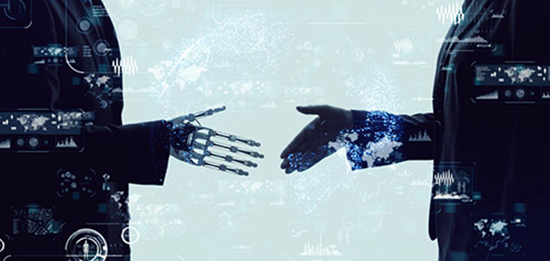
全球增長停滯了,貿易爭端損害著從上海到斯圖加特到西雅圖的制造商們。盡管經濟狀況似乎不妙,但一些最熱衷的支持者們認為工業4.0仍然活躍,發展良好。 工業4.0是一個涵蓋廣泛的概念,它指的是運用大數據、改進的機器人和人工智能系統來運營業務。人們寄望于它,在未來十年乃至更長時間里,成為全球增長的主要驅動力。是的,甚至包括制造業。 到2035年,人工智能賦能的行業,將為全球經濟創造14萬億美元的價值,這是咨詢業巨頭埃森哲的預測。 這一估測,具體來說是來自于埃森哲實驗室的全球高級執行董事馬克·卡萊爾·比利爾德,他在周三參加阿姆斯特丹的人工智能世界峰會作主題演講時,輕松地說出了這一數據。他還舉了實例,有研究追蹤了一項在人工智能賦能自動化領域增長迅速的業務:呼叫中心。五年前,人工智能機器人可以成功解決十分之一的客戶電話,而如今這個數字是60%。 另外他還預測說,這一自動化的推進,不會像一些悲觀的經濟學家擔心的那樣,消滅很多人類的工作機會。 但是,在科技人士慶祝一個階段的成功之前,有一個警告得聽一聽。 卡萊爾·比利爾德說,人工智能不會造成對工作機會的威脅,“因為這些系統并不是很聰明。”人工智能及其各種迭代:機器學習、自然語言處理、機器視覺、音像識別等很好地應用在高度專業的任務中。在一些方面它干得不錯,比如預測明天的天氣、訂購電影票、晚高峰時幫你找出最快的回家線路等等。各種商業活動越來越多地使用企業級人工智能,從搜集到的巨量的有結構和無結構的數據中尋找出意義,剔除低效并節省成本。 但卡萊爾·比利爾德也提到,人工智能還是有盲點。它被訓練出解讀特定數據庫的能力,卻不能從復雜世界里引導出意義或者背景知識。人工智能是專家,卻不是雜家。因此,要讓這些系統真正智能化,還有許多工作要做。 紐約大學的心理與神經科學教授、《重啟人工智能》(Rebooting AI)一書的作者加里·馬庫斯,作了一番更加坦白的評估。他稱深度學習——即人工智能的一個分支,僅需少量甚至無需人類的監管就能處理巨量數據——是一個錯誤的命名。它適用于一些狹義特定的項目,但就其大被吹噓的潛力,馬庫斯表示質疑,比如它能改革交通系統(自動駕駛汽車)和醫療系統(分析巨量的核磁共振圖像來找出癌癥擴展的跡象)嗎?馬庫斯說,“深度學習不代表深度理解。” “有多少放射科醫生被深度學習系統替代了?”他自問自答,“一個也沒有。” 卡萊爾·比利爾德認為,要使得人工智能系統真正有效,需要把它們設計成負責任的、具有透明度的以及沒有偏見的——而不只是干活超級快的小兔子。只有那時,人工智能系統才能達成其所有的潛力。 在人工智能世界峰會的首日,許多先期的探討都是關于建立所謂具有倫理的人工智能系統的必要性。馬庫斯和卡萊爾·比利爾德,與其他人一樣,也敦促開發界要構建出負責任的、透明的、不帶偏見的人工智能系統。 卡萊爾·比利爾德說,除非它是負責任的,“否則沒有人會信任它,也沒有人會使用它。”(財富中文網) ? 譯者:宣峰 |
Global growth is stalling. Trade wars are hammering manufacturers, from Shanghai to Stuttgart to Seattle. But, awful as today’s economic outlook appears, Industry 4.0 is alive and well, its most ardent backers say. Industry 4.0 is the catch-all term for the implementation by businesses of big data, improved robotics and artificial intelligence systems. And it’s still expected to be a major driver in global growth over the next decade, and beyond. Yes, even in manufacturing. By 2035, this A.I.-powered push will provide a $14 trillion boost to the global economy, consulting giant Accenture predicts. That’s the assessment of Marc Carrel-Billiard, global senior managing director at Accenture Labs, who rattled off these numbers during his keynote presentation at World Summit A.I. in Amsterdam on Wednesday. By way of example, he cited research that traced the progress in one growing area of A.I.-powered automation: call centers. Five years ago, A.I. bots could successfully resolve one out every ten customer phone calls. Today, he said, it’s 60%. Moreover, he predicted, this push to automate will not be the jobs-killer the more bearish economists out there fear. But before technologists take a victory lap, there’s a caveat. They’re not a threat to jobs, he says, “because these systems are not very intelligent.” AI—and its many iterations: machine learning, natural language processing, machine vision, image- and voice-recognition—is well adapted at highly specialized tasks. It does a decent job telling you what the weather will be tomorrow, or ordering movie tickets or helping you find the fastest route home during the evening commute. All manner of businesses are using A.I. increasingly on the enterprise level to make sense of the vast flows of structured and unstructured data they collect to root out inefficiencies, and save costs. But, as Carrel-Billiard notes, A.I. still has a blind spot. It’s trained to interpret certain data sets, not infer meaning or context from a complicated world. A.I. is a specialist, not a generalist, he says. And therefore, much work is needed to make these systems truly intelligent. Gary Marcus, professor of psychology and neural science at New York University and author of Rebooting AI, is even more frank in his assessment. He calls deep learning— the subset of A.I. that can make sense of huge amounts of data with little to no oversight from human minders—a misnomer. It’s good at narrowly focused tasks, but he questions its much-ballyhooed potential to, for example, revolutionize transportation (self-driving cars) and medicine (analyzing huge volumes of MRI scans for signs of cancerous growths). “Deep learning is no substitute for deep understanding,” he says. “The number of radiologists who’ve been replaced by deep-learning systems?” he asks. “Zero.” Carrel-Billiard, for one, believes that in order for A.I. systems to be truly effective they need to be designed to be accountable, transparent and free of bias—not just super-fast task rabbits. Only then will such systems reach their full potential. On day one of the World Summit A.I., much of the early discussion was about the need to build so-called ethical A.I. systems. Marcus and Carrel-Billiard, among others, challenged the development community to build A.I. systems that are accountable, transparent and free of bias. Unless it’s responsible, Carrel-Billiard says, “nobody will trust it, and nobody will use it.” |
?